Modeling spatial and temporal dynamics in yield with crop modeling and remote sensing
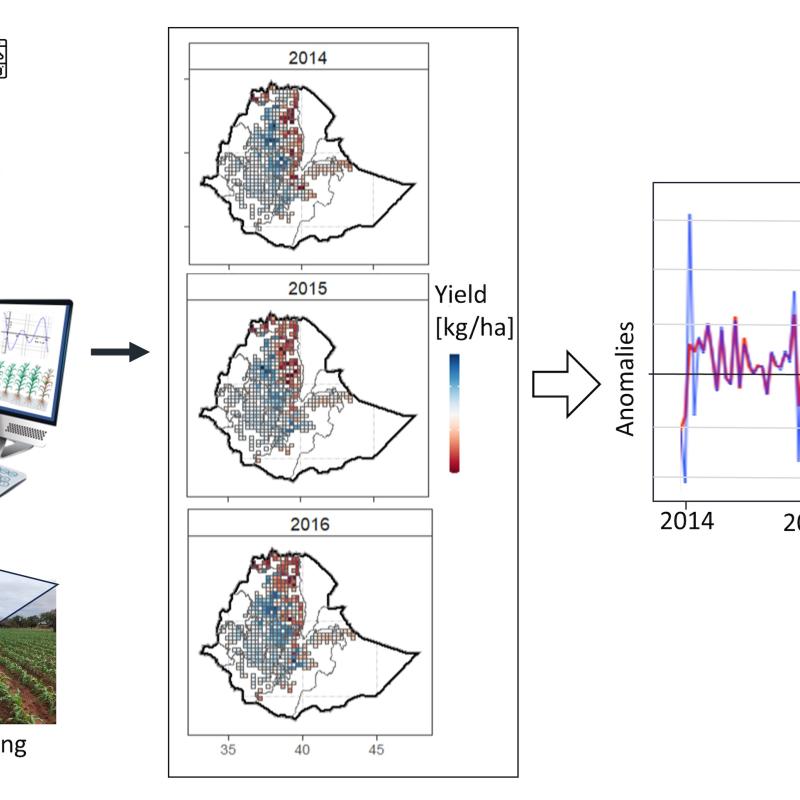
The topic is also suitable for GEM students in track 3 – GEM for Ecosystems & Natural Resources.
Suggested electives: Quantitative remote sensing of vegetation parameters
Food insecurity is a big issue in eastern and southern Africa and projections are that this is going to get worse with climate change and variability. There are many drivers of yield variability such as weather, soil fertility, crop pests and management, all of which vary in both space and time. Providing reliable yield estimates from remote sensing data has been a subject of research interest for a long time. However, remote sensing provides empirical relationships between crop yield/growth with remote sensing data and therefore coupling this with crop growth models enables a more detailed understanding of the yield formulation within a season that explain the outcome. With that understand the best crop management strategies and better forecasting can be developed for the future to avert famines and associated societal outcomes such as migration, land degradation and conflicts. Therefore, in this research project you will select to setup a gridded crop model for a country in Eastern or Southern Africa and estimate crop yields over time by comparing with reference yield data sources. After that, you will find the best remote sensing products that are connected to the simulated yields and develop an explainable model for subnational yield from remote sensing at country level that can be used for (i) within season forecasting of yield and (ii) identifying the most limiting factors for yield that can act as entry points for intervention.
This project aims to develop and apply an approach for estimating yield of major food crops in using gridded crop modeling and time series remote sensing data in in Eastern and Southern Africa. Using DSSAT/APSIM you will calibrate and run a spatialized crop simulation model at country level and estimate yield at grid level for maize and/or sorghum and compare the crop growth and yield from the model with time series remote sensing data. The project aims to provide an understanding of the processes involved in yield formulation and how these can be captured by remote sensing data products such as LAI and vegetation indices. The overall goal is to develop a scalable tool for yield monitoring at subnational level that can be used for tech-powered climate-smart agriculture design in the region (and transferable).
This topic is perfect for SE because it is interdisciplinary and involves the development and application of key technical skills in climate (big) data processing and modeling that are important for real-life decision making. The developed modeling and data processing pipelines will be key asserts for further application of knowledge in decision making and solving problems. The students will also be able to compile the project into a portfolio that they can use for future reference to showcase their capacity to deal with different types of data to solve a problem.