Human intelligence, ChatGPT and geoscience footprints
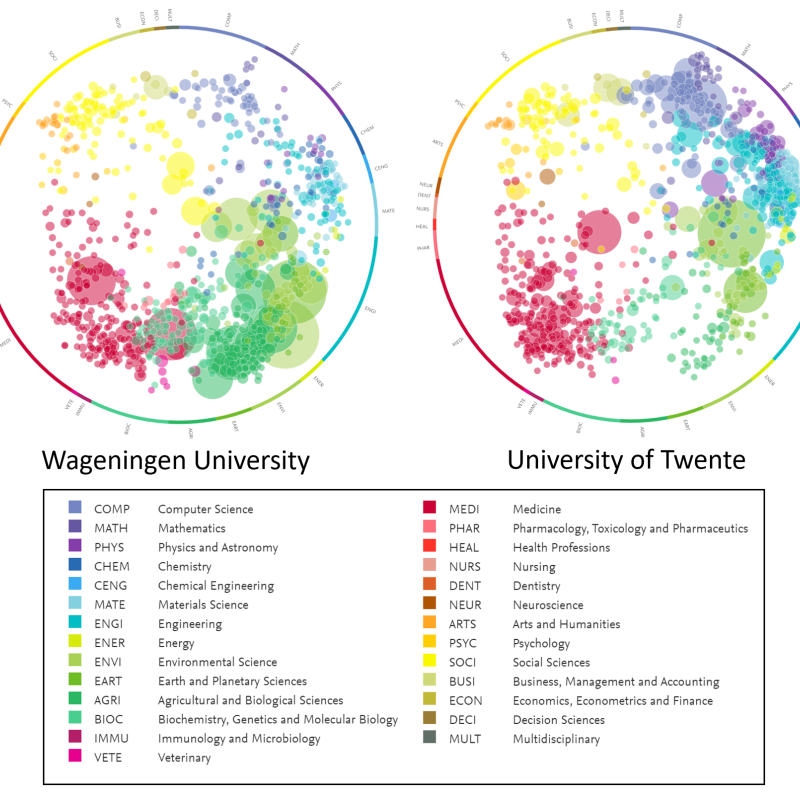
Individuals and the organisations in which they work need to cooperate in projects and research to withstand an increasing competition. To find the right partners, it is essential know and match the right expertise (human intelligence). This can be done through footprints. Footprints refer to knowledge footprints (aka knowledge maps, or profiles) of organisational and personal expertise which are created for the purpose of representing, sharing and promoting scientific and professional capabilities amongst organisations and people. Such profiles can be found on platforms such as Google Scholar and LinkedIn, but they lack the focus and details of specific domains, such as geoscience. Moreover, the visualisations, representing this human intelligence differ per tool. Figure 1 shows the general footprints (based on all Elsevier literature) for Wageningen university and University of Twente in the SciVal tool.
The objective of this MSc topic is to devise a method to visualise expertise of people in the specific domain of geoscience. The expertise can be represented by a combination of concepts (small building blocks of knowledge) from a Body of Knowledge (BoK) of geoscience. This BoK contains two parts: 1. A BoK that is derived from literature published by the person/organisation and 2. an already existing BoK, covering the geoscience domain (Lemmens et al., 2022, see also http://www.eo4geo.eu/bok/). These two BoKs can be connected via AI. The student will use ChatGPT (OpenAI, 2023) to do this. The resulting connections need to be visualised in a concept map, which will serve as knowledge footprint of that person/organisation. You will analyse and test visualisation tools that are suitable for creating and using these visualisations for sharing these on a web page. Examples of those are AI-expert finder https://www.utwente.nl/en/digital-society/research/data/ai-experts/?tab=graph, the EO4GEO Occupational Profile Tool (see Figure 2), the Living Textbook https://www.itc.nl/facillities/living-textbook/ (with the new Dotron visualiser plug-in) and other tools.
Elsevier (2023) SciVal, Strategic insights to help your research programs thrive. https://www.elsevier.com/solutions/scival?dgcid=RN_AGCM_Sourced_300005487.
Huang, Y., Glänzel, W. & Zhang, L. Tracing the development of mapping knowledge domains. Scientometrics 126, 6201–6224 (2021). https://doi.org/10.1007/s11192-020-03821-x
Lemmens, R., Albrecht, F., Lang, S., Casteleyn, S., Stelmaszczuk-Górska, M., Olijslagers, M., Belgiu, M., Granell, C., Augustijn, E., Pathe, C., Missoni-Steinbacher, E., & Monfort muriach, A. (2022). Updating and using the EO4GEO Body of Knowledge for (AI) concept annotation. AGILE: GIScience Series, 3, 1-6. https://doi.org/10.5194/agile-giss-3-44-2022
OpenAI (2023) Introducing ChatGPT. https://openai.com/blog/chatgpt