Critical nodes for food security: detecting food storage facilities using remote sensing images
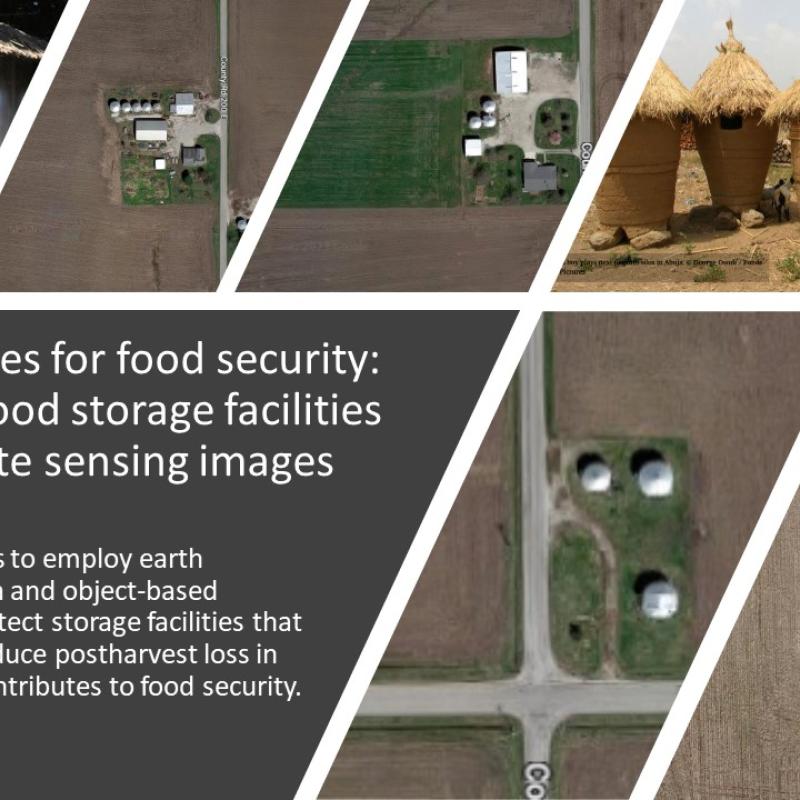
The topic is open for also suitable for GEM students in track 1 – GEM for Urban-Rural Interactions.
The topic is open for also suitable for GEM students in track 3 – GEM for Ecosystems & Natural Resources.
Suggested elective: Advanced Image Analysis
Photo credit to: https://ypard.net/resources/blog/when-food-storage-can-become-food-security under CC license.
Postharvest losses are a main threat to food security, which is a measurable waste of the edible share of food that is available for consumption but is not consumed by people for whatever reason. In Eastern and Southern Africa, the loss is estimated to be 1.6 billion USD annually. This is particularly critical for smallholders in less-developed regions, causing food and nutrition insecurity and unwanted economic losses. The causes of postharvest losses are diverse and studies have found that improving storage facilities can significantly reduce the possibilities of pests and fungus ensuring household food security and rural livelihoods. Better storage also allows farmers to dry grains and use them for emergencies or sell them in a favorable price position.
However, our knowledge of these critical, farm-level storage facilities is very limited. The spatial coverage and capacity of these facilities are not known to researchers and policymakers, leaving a gap in food security relevant policies.
This project aims to employ earth observation data and object-based algorithms to detect storage facilities that are critical to food security. The possibility of exploiting open access very high spatial resolution data (such as google satellite images) and high spatial resolution data (such as Sentinel-2 data) will be investigated. The main activities of this thesis are:
- Database Preparation: prepare the database of training data by photo-interpretation of high-resolution Google images in the United States, where food storage facilities can be easily located.
- Food Storage Facilities Detection: defining an approach for the automatic detection of food storage facilities. A benchmark of object-based detection algorithms will be explored to identify the best solution.
- Evaluation of the Approach: test the generalization capability of the developed approach by testing it in different countries, i.e., Brazil, African countries.
Gitonga, Z. M., de Groote, H., Kassie, M., & Tefera, T. (2013). Impact of metal silos on households’ maize storage, storage losses and food security: An application of a propensity score matching. Food Policy, 43, 44–55. https://doi.org/10.1016/j.foodpol.2013.08.005
Luo, Y., Huang, D., Wu, L., & Zhu, J. (2022). The impact of metal silos on rice storage and storage losses in China. Food Security, 14, 81–92. https://doi.org/10.1007/s12571-021-01194-4/Published
We could reduce the transferability of the model.