Characterisation of ice subsurface structures using radar sounder data and machine learning methods
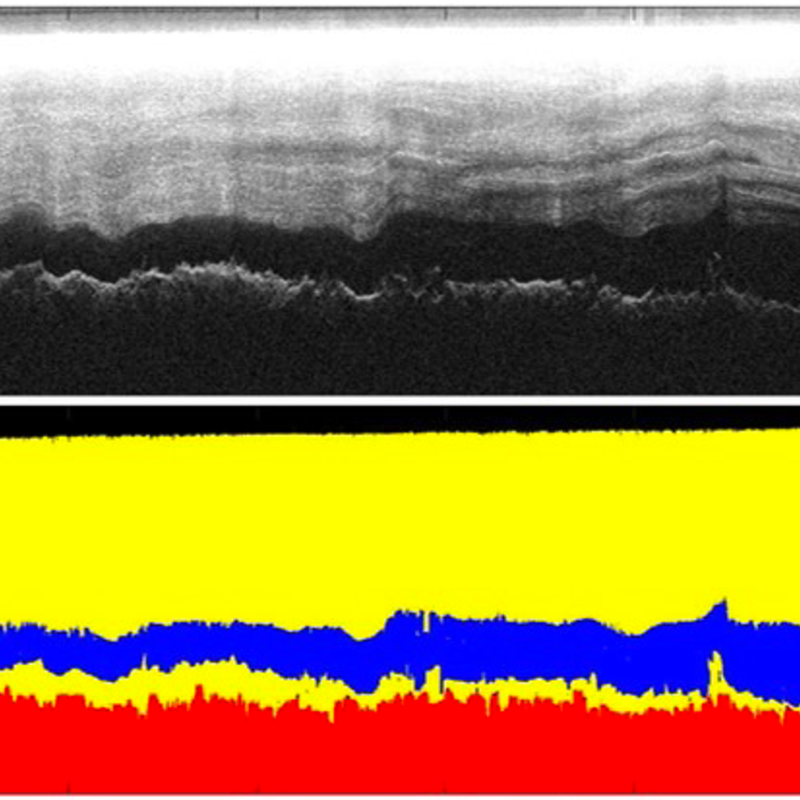
The Antarctic ice sheet covers almost the whole area of the Antarctic continent. It is the largest ice mass on Earth, with an average ice thickness of about 2 kilometers [1]. Global warming has shown a noticeable impact on the loss of ice in this ice sheet. According to ice mass measurements by NASA's GRACE satellites, Antarctica is melting at an average rate of about 150 billion tons per year [2]. This is extremely threatening because it is a major contributor to sea level rise, causing devastating effects on coastal cities and low-lying areas around the world like the Netherlands.
Analysis of the ice sheets' subsurface provides valuable information about ice dynamics, which is essential for understanding how ice sheets respond to climate change. A radar sounder (RS) is a nadir-pointing instrument designed for imaging and probing the subsurface of ice sheets. They work by emitting a low-frequency radar signal that penetrates the ice and returns to the instrument. The instrument measures the power reflected by subsurface structures (such as layers and bedrock). The RS’s measurements are often recorded in 2-D matrices (i.e., images) called radargrams. RSs have been largely applied in studying the Antarctica ice sheet during several dedicated airborne campaigns [3].
Ice subsurface structures in radargrams are distributed in both space (azimuth) and depth (range) [4], [5]. This represents a challenge in the development of machine learning (ML) methods for the identification and characterization of these structures. This topic aims at the development of an ML technique to simultaneously consider space and depth in RS data. This study explores the application of such a technique for analyzing and the complete characterization of spatial and depth-associated distributed patterns of structures in the Antarctica subsurface.
[1] https://www.nsf.gov/geo/opp/antarct/science/icesheet.jsp
[2] https://climate.nasa.gov/vital-signs/ice-sheets
[3] https://data.cresis.ku.edu/data/rds/
[4] A. -M. Ilisei and L. Bruzzone, "A System for the Automatic Classification of Ice Sheet Subsurface Targets in Radar Sounder Data," in IEEE Transactions on Geoscience and Remote Sensing, vol. 53, no. 6, pp. 3260-3277, June 2015, doi: 10.1109/TGRS.2014.2372818.
[5] A. -M. Ilisei, M. Khodadadzadeh, A. Ferro and L. Bruzzone, "An Automatic Method for Subglacial Lake Detection in Ice Sheet Radar Sounder Data," in IEEE Transactions on Geoscience and Remote Sensing, vol. 57, no. 6, pp. 3252-3270, June 2019, doi: 10.1109/TGRS.2018.2882911.