Timely assessment of crop phenology through the integration of Sentinel-2 imagery and crowdsourced photographs
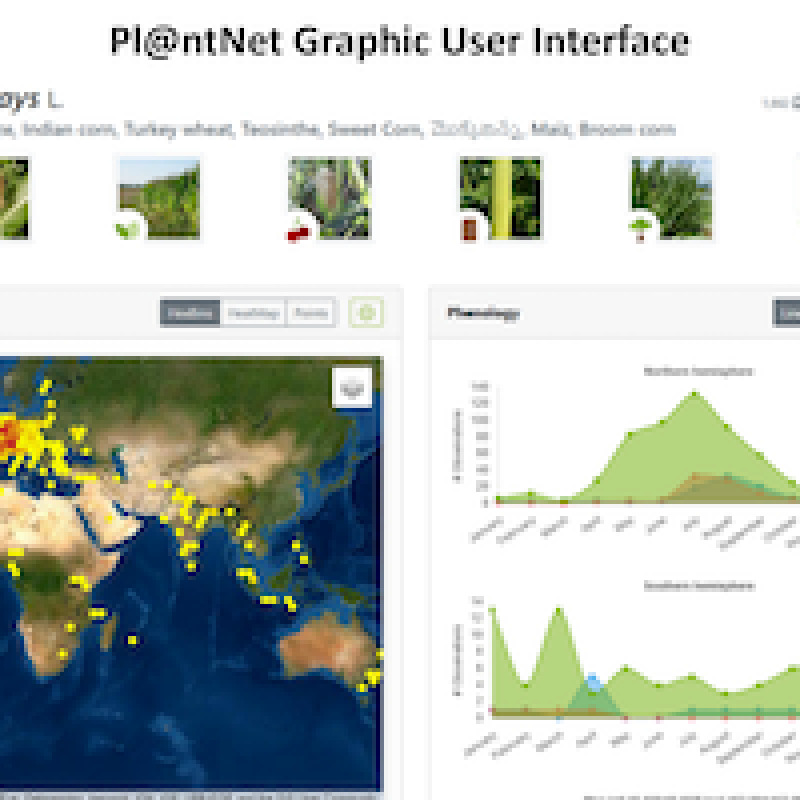
- Good programming experience is a prerequisite.
- The topic is open for also suitable for GEM students in track 3 – GEM for Ecosystems & Natural Resources.
Increasingly frequent extreme weather events have a major impact on agriculture and pose a threat to sustainable food production. The impact of an extreme event on yield depends on its timing relative to the phenological stage of the crop. Therefore, systems for timely monitoring of crop phenology are needed. With the recent availability of high-resolution multispectral Sentinel-2 data, acquired globally with a short revisit time, it is possible to accurately monitor crop phenology. Although in situ data collection is critical to develop and validate effective crop phenology assessment over large areas, coordinated and repeated field campaigns to collect such information are scarce. Crowdsourced data collection, including through geo- and time-tagged photographs, has the potential to fill this gap. The purpose of this study is to test whether information extracted from a large database of crowdsourced photographs collected through the smartphone application Pl@ntNet can be used for timely monitoring of crop phenology over large areas.
This MSc thesis aims to explore the possibility of using heterogenous data sources to monitor crop phenology. Information provided by Sentinel-2 time series will be combined with that provided by crowdsourced photographs collected through the Pl@ntNet smartphone application to distinguish specific phenological stages, principally flowering and fruiting. The main activities of this thesis are:
-
Data Collection: prepare the database of time-stamped geo-tagged Pl@ntNet crowdsourced photographs and Sentinel-2 images. The database will be prepared at European level.
-
Data Preparation Get average flowering/fruiting dates at local scale (e.g., 10km x10 km) and link that with phenological information derived from Sentinel-2.
-
Crop Phenology Monitoring: develop a system for automatically estimating the flowering/fruiting dates using the Sentinel-2 data. A benchmark of machine learning methods will be tested to identify the best solution.
van der Velde, Marijn, et al. "Pl@ ntNet Crops: merging citizen science observations and structured survey data to improve crop recognition for agri-food-environment applications." Environmental Research Letters (2022).