Deep learning-based generation of digital surface model (DSM) using a single high-resolution SAR image
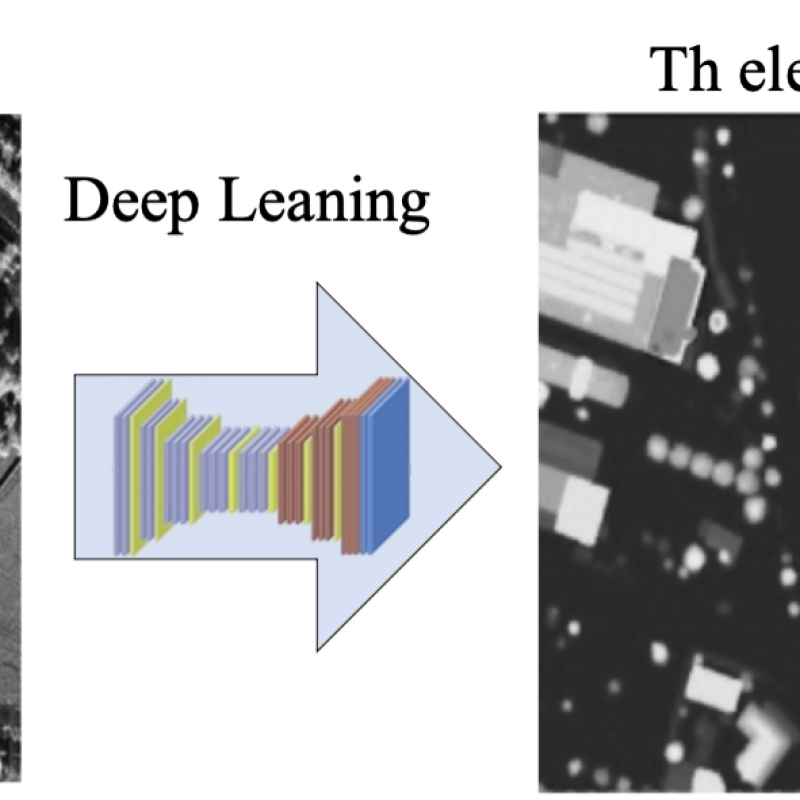
Downward-looking satellite sensors are used to display our three-dimensional (3D) world with 2D remote sensing imagery. Currently, investigations are still underway to develop an advanced technique for estimating elevation models from a single image. Recent and advanced data-driven techniques have shown that height estimation from optical data is possible. However, it turns out that in average half the world is either at night time or is cloudy. Combining these two factors, about 75 percent of the globe is going to be cloudy, nighttime, or both at any given time. This problem urges the use of synthetic aperture radar (SAR) data to reconstruct the elevation model. In this context, the objective of this study is to explore a Deep Learning based technique to reconstruct DSM using a single intensity SAR image.
The main goal of this proposal is to use a convolutional neural network to map a single SAR image into an elevation model. A set of RADARSAT-2 images and LIDAR based DSMs are available to train the network. Thus, the trained model can be used later to build DSM of any area on Earth. Currently, several deep learning solutions have been proposed in the literature for remote sensing image processing. These data-driven methods usually define different network architectures while use a similar cost function (usually RMSE). Such methods may reach their limits and show poor performance in complex regions, such as edge and boundary areas. Thus, the idea is to define a multi-objective cost function to achieve the best performance in different regions. The initial solution can be achieved with the network proposed in the following paper.
Recla, M., & Schmitt, M. (2022). Deep-learning-based single-image height reconstruction from very-high-resolution SAR intensity data. ISPRS Journal of Photogrammetry and Remote Sensing, 183, 496-509.
S. Vitale, H. Aghababaei, G. Ferraioli, V. Pascazio and G. Schirinzi, "A Multi-Objective Approach for Multi-Channel SAR
Despeckling," 2021 IEEE International Geoscience and Remote Sensing Symposium IGARSS, 2021, pp. 419-422, doi: 10.1109/IGARSS47720.2021.9553262.
.