Classification of Sentinel-1 images at subpixel level using deep learning
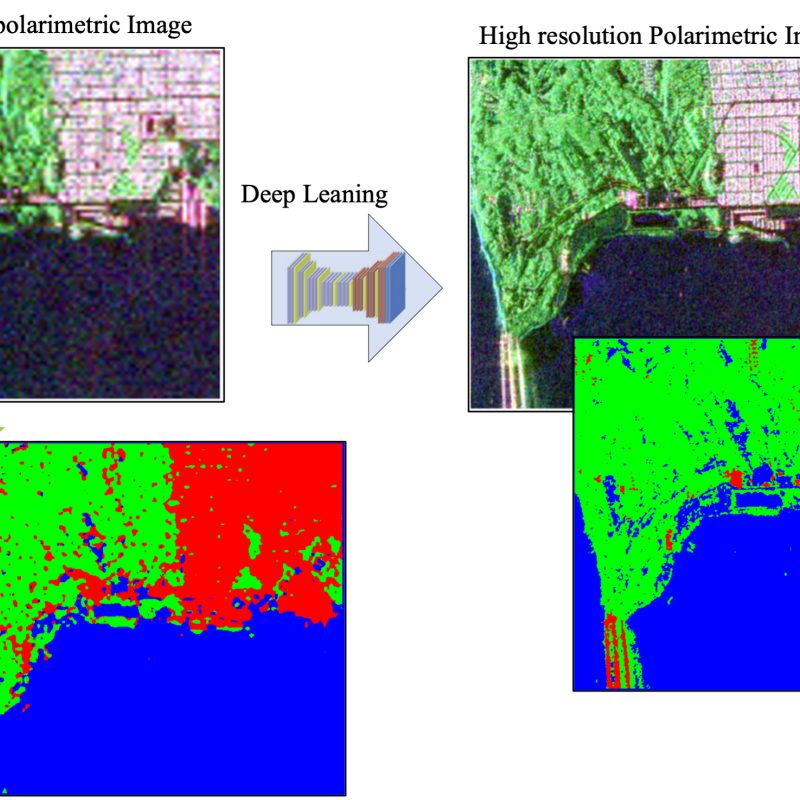
Sentinel-1, with a constellation of radar satellites, operates day and night, providing freely accessible, consistent, and time series SAR images of every part of the Earth. These images contain a wealth of information for Earth inspection and environmental studies and have been used extensively for various applications such as land cover classification, interferometric based elevation/displacement mapping, etc. The low spatial resolution of Sentinel-1 images (e.g., 20 meters in EW mode), however, can limit their applications. Improving the spatial resolution of Sentinel-1 is a prerequisite for extracting depth information in various applications. Therefore, for the first time, this proposal aims to enhance the spatial resolution of Sentinel-1 images and evaluate their efficiency in some applications such as classification.
The main goal of this proposal is to use a Deep Learning technique to reconstruct a finer resolution image from a coarser one. The basic hypothesis is that the low resolution images can be considered as convolved versions of the high resolution images. Therefore, the inversion process can be performed using Deep Learning to convert a coarse resolution image into a finer reconstructed image. Besides choosing the right network architecture, the challenge is to define an efficient loss function for the training process. The idea is to develop a subpixel mapping method that generates a high-resolution image from low-resolution polarimetric SAR data, where the classified image from the output of the network resembles the classified image from reference or actual high-resolution data.
Liao, Z., He, B., & Quan, X. (2020). Potential of texture from SAR tomographic images for forest aboveground biomass estimation. International journal of applied earth observation and geoinformation, 88, 102049
S. Vitale, H. Aghababaei, G. Ferraioli, V. Pascazio and G. Schirinzi, "A Multi-Objective Approach for Multi-Channel SAR
Despeckling," 2021 IEEE International Geoscience and Remote Sensing Symposium IGARSS, 2021, pp. 419-422, doi: 10.1109/IGARSS47720.2021.9553262.
.